Soil moisture mapping using Landsat data in a frigid glaciolacustrine landscape with agricultural production
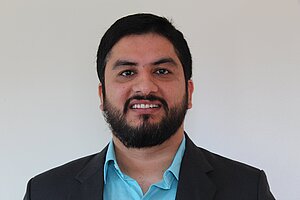
Umesh Acharya is a Ph.D. graduate student in the Department Soil Science, School of Natural Resources Sciences at North Dakota State University (NDSU). He holds two master’s degrees, first one in soil science from NDSU (2016-2018) and second in Horticulture from Tamil Nadu Agricultural University, India (2014-2016). He did his undergraduate degree (Bachelor of Science in Agriculture) from Tribhuvan University (2007-2011) and have worked in Non-governmental organizations for three years before joining the Department of Soil Science at NDSU. His current research is focused on using Landsat 8 satellite images, weather station data and soil characteristics to predict field soil moisture. The goal of his work is to develop a model to predict soil moisture using machine learning algorithms and satellite images.
Soil moisture mapping using Landsat data in a frigid glaciolacustrine landscape with agricultural production
Soil moisture (SM) is an important variable in hydrology and climate studies and has been an important factor in water infiltration, runoff, evaporation, heat and gas exchange, and erosion processes. Weather stations are easy sources for getting SM, rainfall, evapotranspiration that provide estimates over large crop fields. There is a need to develop a model, which can extrapolate soil moisture values around weather stations with crop fields based on types of crops, growth stage, soil texture and crop residue. Rainfall is a major contributor for change in moisture content of soil followed by snowfall, irrigation, and rise in water table. There is insufficient research to show the relation between amounts of rainfall to SM content around weather stations and crop fields around Red River Valley of North. It will give an idea how much rainfall is enough to bring soil moisture to optimum growing condition. The use of remote sensing technology has achieved a varying degree of success in frequently mapping soil properties over large areas. Soil moisture estimation using satellite images needs information on the dynamic nature of actual field circumstances and micrometeorological variability in real time. There is a need for better spatial estimates of surface SM to improve forecasting of the impacts from drought and floods as well as climate projections.
Project Objectives
The specific research objectives are:
- To determine the level of discrepancies in soil moisture between weather stations in the Red River Valley of the North (RRVN) and nearby agricultural fields. (completed)
- To determine the duration of temporal dependency of these soil moistures to recent rainfall and evapotranspiration rates. (completed)
- To predict soil moisture from the Landsat 8 satellite images using Optical Trapezoid Model (OPTRAM). (in progress)
Progress:
Soil moisture prediction using weather station
Soils were collected from 75 plots over a period of 4 months (June to September) for the 2019 growing season from a 16 days interval around 25 weather stations installed on either side of Red River of North. Those soil samples were processed and bulk density, volumetric water content, saturated hydraulic conductivity, percent sand, silt and clay were calculated in the lab. The crop types and their growth stages and crop residue content were noted every time field pots were visited for soil sampling. Rainfall and potential evapotranspiration were downloaded online from the North Dakota Agricultural Weather Station (NDAWN) site for each station under the study. Soil moisture of nearby fields was predicted using soil moisture at the weather station using a linear regression equation. The discrepancy in the soil moisture between field and weather station under different crop type, residue content, soil texture was determined by linear regression equation. Similarly, temporal dependency of these soil moisture with recent rainfall and PET was determined using nonlinear regression equation. Based on the first two objectives a manuscript is ready for submission to the journal for publication.
Soil Moisture prediction using Optical Trapezoid Model (OPTRAM)
Landsat 8 satellite images were used to develop soil moisture map using OPTRAM model. We have used Google earth engine software to prepare OPTRAM soil moisture maps. In-situ soil moisture was compared with the corresponding values of the OPTRAM soil moisture and weak correlation was observed at the initial stage of the study. We are in the process of using a machine learning model that uses OPTRAM values, four-day cumulative rainfall, percent clay and standardized precipitation index as predictor variables. Third objective is in progress.
Significance:
Weather stations provide a lot of information (temperature, wind speed and direction, evaporations) and have huge potential to predict soil moisture of the nearby field. There are 25 weather stations around the Red River Valley and can be used for soil moisture prediction with additional field information (soil type, crop and their growth stages). The proposed study showed how much discrepancies soil and crop characteristics can create in predicting field soil moisture using weather station data. In addition, this research used Landsat 8 satellite images to predict soil moisture as a non-destructive method and can cover large spatial areas. This research provides a whole new approach of soil moisture prediction using weather station, satellite image and machine learning model.
Conference/Seminar Presentations:
Acharya U., A.L.M. Daigh and P.G. Oduor. 2020. Soil moisture mapping using Landsat data in a frigid glaciolacustrine landscape with agricultural production. Translating visionary science to practice, 2020 ASA-CSSA-SSSA International Annual Meeting, November 9-13, Virtual.
Acharya U., A.L.M. Daigh and P.G. Oduor. 2021. Use of Landsat 8 Image and Meteorological Data to Map Soil Moisture in the Red River Valley. The Soils and Crops Conference, March 16-17, 2021, University of Saskatchewan, Canada
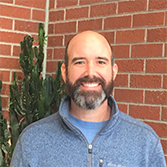
Aaron Daigh
Asst Prof/Soil Phys-School Nat Res AES School of Nat Res Sciences
aaron.daigh@ndsu.edu
Office: Walster 147
Phone: 701-231-8354